Naive Bayes Algorithm Explained .
Naive Bayes classifier is a probabilistic machine learning model thatโs used to solve classification problems , is actually based on the Bayes theorem.
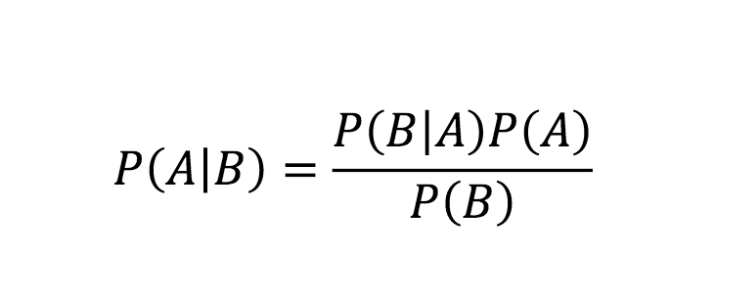
The Naive Bayes Model is defined as follows :
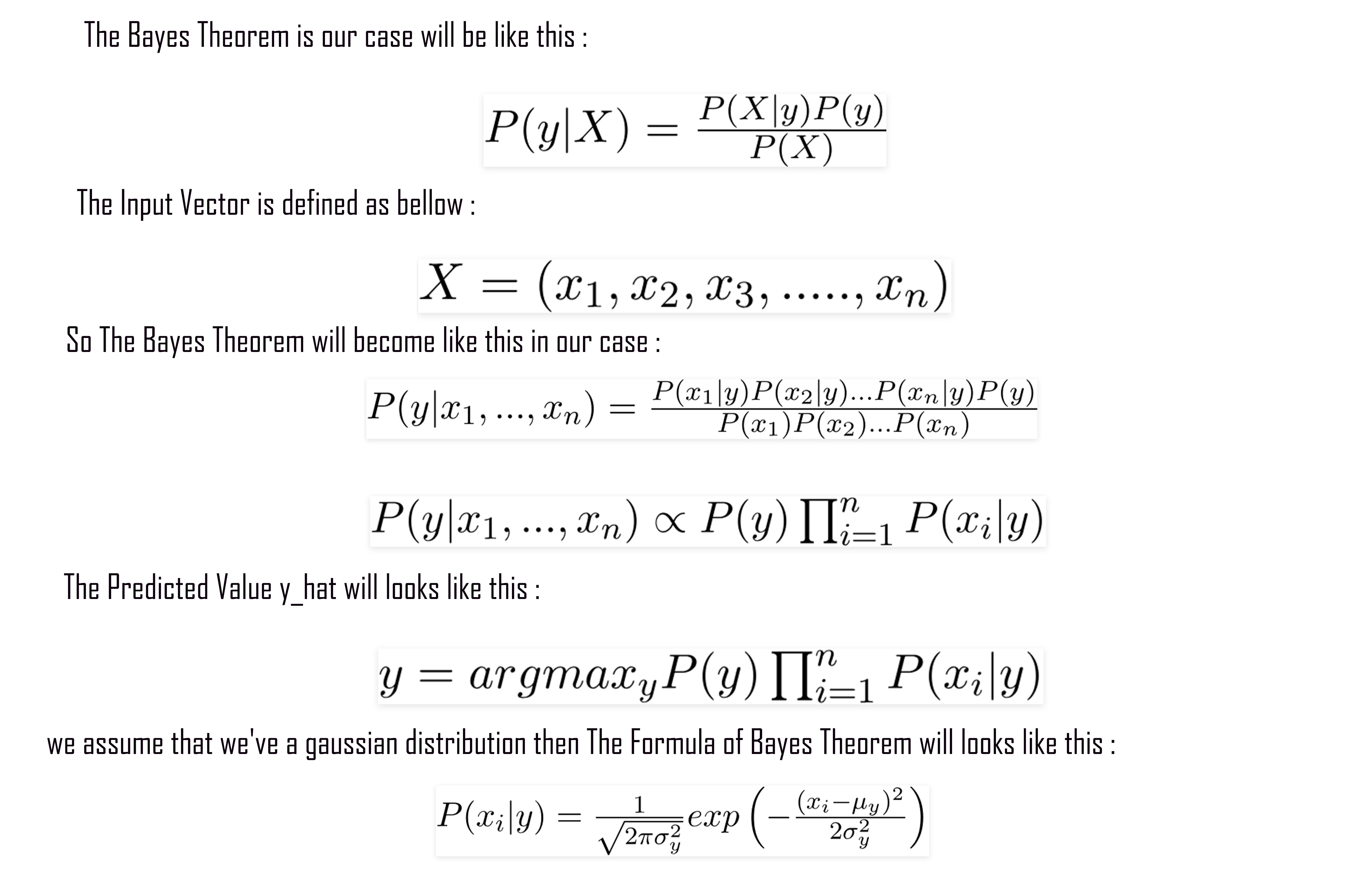
Implementation :
1
2
3
4
5
6
7
8
9
10
11
12
13
14
15
16
17
18
19
20
21
22
23
24
25
26
27
28
29
30
31
32
33
34
35
36
37
38
import numpy as np
from sklearn.datasets import make_classification
from sklearn.model_selection import train_test_split
from sklearn.metrics import accuracy_score
class NaiveBayes:
def fit(self , x , y):
self.classes = np.unique(y)
self.nbr_classes = len(self.classes)
self.mean = np.zeros((self.nbr_classes , x.shape[1]) , dtype = np.float64)
self.variance = np.zeros((self.nbr_classes , x.shape[1]) , dtype = np.float64)
self.probability_of_a_Class = np.zeros(self.nbr_classes , dtype=np.float64)
for index , classValue in enumerate(self.classes):
class_i = x[classValue == y]
self.mean[index , :] = class_i.mean(axis = 0)
self.variance[index , :] = class_i.var(axis = 0)
self.probability_of_a_Class[index] = float(class_i.shape[0] / y.shape[0])
def predict(self , x):
y_predicted = [self.predictOneSample(xi) for xi in x]
return np.array(y_predicted)
def predictOneSample(self , x):
probabilities = []
for index , classValue in enumerate(self.classes):
probability = np.log(self.probability_of_a_Class[index]) + np.sum(np.log(self.probability_Density_function(index, x)))
probabilities.append(probability)
return self.classes[np.argmax(probabilities)]
def probability_Density_function(self , classIndex , x):
mean = self.mean[classIndex]
variance = self.variance[classIndex]
numerator = np.exp( - (x - mean**2) / (2 * variance**2))
denominator = np.sqrt(2 * np.pi * variance)
return numerator / denominator
Testing The Model :
1
2
3
4
5
6
7
8
9
10
11
x , y = make_classification(n_samples=1000 , n_features=10 , n_classes=2 , random_state=0)
x_train , x_test , y_train , y_test = train_test_split(x,y,test_size=0.25)
N_Bayes = NaiveBayes()
N_Bayes.fit(x_train, y_train)
y_hat = N_Bayes.predict(x_test)
accuracy = accuracy_score(y_test , y_hat)
print("Model Accuarcy : ", accuracy)